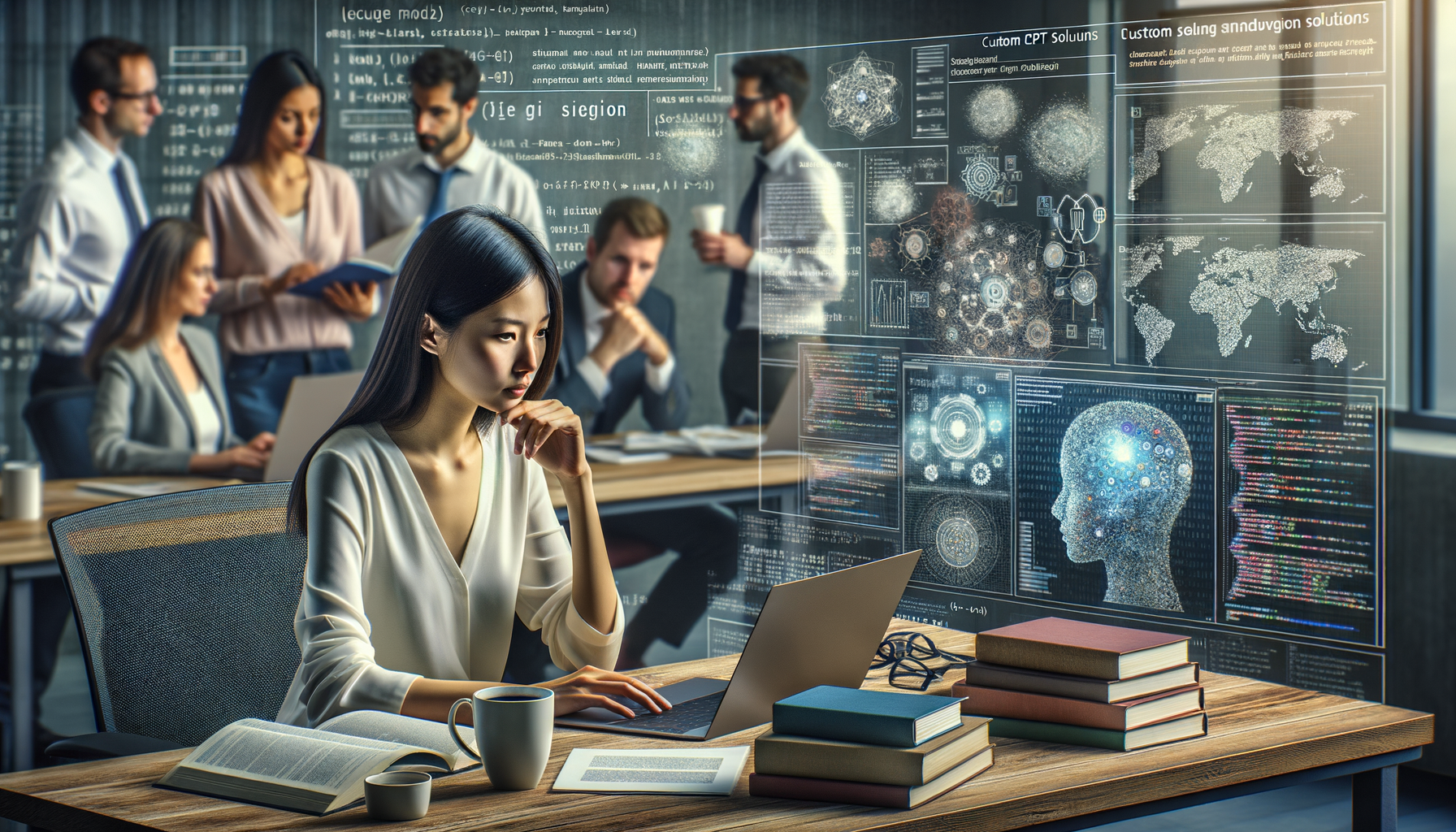
In the fast-paced world of artificial intelligence, staying ahead means constantly evaluating the tools at our disposal.Â
Imagine a world where every language model you deploy not only meets but exceeds your expectations in terms of accuracy and efficiency. This isn’t just a dream—it’s becoming a reality with custom GPT solutions.Â
Benchmarking these models isn’t just about numbers; it’s about understanding their potential to transform industries. From healthcare to finance, custom GPT models are setting new standards.Â
In this blog post, we’ll dive deep into how benchmarking custom GPT solutions can offer unprecedented insights and drive significant advancements in AI applications. Join us as we explore the cutting-edge of language model technology and its impact across various sectors.
The Importance of Benchmarking in AI Development
Benchmarking isn’t just a technical necessity; it’s the heartbeat of AI development. Think of it as the compass that guides AI innovations.Â
Without benchmarking, we’re essentially navigating in the dark. It helps us understand where a model excels and where it falls short. This clarity isn’t just about improving what we have; it’s about inspiring new ideas that push the boundaries of what AI can do.Â
By comparing different models, like those created with CustomGPT.ai, we pinpoint exactly how and where improvements can be made. This isn’t just beneficial—it’s crucial for any serious AI development, ensuring that we’re not just making changes, but making the right changes.
Overview of Custom GPT Solutions
Custom GPT solutions are revolutionizing how we approach AI-driven interactions. Imagine crafting a chatbot that not only responds accurately but also reflects your brand’s unique voice without a single line of code. That’s the power of CustomGPT.ai.Â
With its no-code visual builder, you can design and deploy AI chatbots tailored to your specific needs. This platform stands out by offering features like anti-hallucination and automatic citation, ensuring that your chatbot delivers reliable and verifiable information.Â
Whether you’re looking to enhance customer service, streamline operations, or offer personalized user experiences, CustomGPT.ai provides the tools to make it happen efficiently and effectively.
Understanding Language Models
Did you know that the first language model was developed over half a century ago? Yet, it’s only in recent years that their capabilities have skyrocketed, thanks to advancements in AI.Â
Language models, like the ones we use in CustomGPT.ai, are not just about understanding or generating text. They are the backbone of how machines interpret human language, turning gibberish into meaningful conversations. In this section, we’ll peel back the layers of these complex systems.Â
From their humble beginnings to the sophisticated GPT models we use today, we’ll explore how they learn from vast amounts of data and how this technology is shaping the future of communication. Join me as we dive into the fascinating world of language models.
What are Language Models?
Language models are at the heart of how AI understands and generates human-like text. These models are trained on vast datasets of written language, learning patterns, and nuances that enable them to predict the next word in a sentence.Â
But it’s not just about predicting text; it’s about understanding context, humor, and even sarcasm, making interactions seem as natural as possible. This capability forms the foundation for tools like chatbots, translation services, and content creation aids, transforming how we interact with digital platforms.Â
CustomGPT.ai leverages this technology, allowing anyone to build powerful, context-aware chatbots without a single line of code.
Evolution of GPT: From GPT-1 to GPT-4
The journey from GPT-1 to GPT-4 is a fascinating tale of rapid evolution in AI. GPT-1, launched in 2018, was a pioneer, crafting coherent text but often lacked consistency.Â
Fast forward to 2019, and GPT-2 brought significant improvements with a much larger dataset, enhancing the model’s ability to generate more reliable text. The leap to GPT-3 was monumental, introducing even more sophistication and a broader range of applications, from writing assistance to coding.Â
Now, GPT-4 pushes the boundaries further, promising enhanced accuracy and nuanced understanding, setting new standards for what AI can achieve in natural language processing.Â
This evolution not only showcases technological advancement but also highlights the increasing integration of AI in solving complex, real-world problems.
Setting Up Benchmarks
Imagine you’re a chef trying to perfect a recipe. You’d tweak, taste, and test different ingredients to see what works best, right?Â
Setting up benchmarks for language models isn’t much different. It’s about finding the right “ingredients” — metrics, tools, and tests — to ensure our AI systems perform not just adequately, but exceptionally.Â
In this section, we’ll explore how to set up effective benchmarks that help us understand the capabilities and limitations of language models like those developed with CustomGPT.ai.Â
This process is crucial for pushing the boundaries of what these models can achieve and ensuring they meet the specific needs of their applications. Join me as we delve into the essentials of benchmarking in the AI world.
Key Performance Indicators for Language Models
When setting up benchmarks for language models, it’s crucial to focus on the right Key Performance Indicators (KPIs). Think of these KPIs as the secret sauce that tells you how well your language model is cooking up answers.Â
Accuracy is a no-brainer; it measures whether the model’s responses hit the mark. But there’s more to it. We also look at robustness—how well does the model handle different types of input?Â
And let’s not forget efficiency; it’s all about doing more with less. By honing in on these KPIs, we ensure our language models perform optimally across various scenarios.
Tools and Technologies for Benchmarking
When diving into the world of benchmarking language models, the choice of tools and technologies can make or break your analysis.Â
For starters, using a robust platform like CustomGPT.ai can streamline the process. This tool stands out because of its no-code visual builder, allowing you to set up and run benchmarks without getting tangled in complex code.Â
Additionally, integrating various data sources becomes a breeze, ensuring that your benchmarks are as comprehensive as possible. By leveraging such advanced technologies, you’re not just testing; you’re gaining deep insights that drive better decision-making in AI deployments.
Case Studies
Did you know that real-world applications of language models often reveal insights that lab conditions can’t? That’s where our case studies come into play.Â
In this section, we’ll dive into specific examples of how CustomGPT solutions have been benchmarked in diverse settings like enterprise applications and academic research.Â
These stories not only highlight the versatility and robustness of CustomGPT.ai but also showcase the tangible benefits and challenges encountered during these implementations.Â
Join us as we explore these fascinating case studies, offering a closer look at the practical impact of AI in different sectors.
Benchmarking GPT-3 in Enterprise Applications
When it comes to integrating GPT-3 into enterprise applications, the real-world data speaks volumes. For instance, consider how a leading Customer Service Platform leveraged GPT-3. They set benchmarks to measure response accuracy and speed, crucial for customer satisfaction.Â
The results? A significant reduction in response times and a boost in resolution rates. This case study not only highlights the efficiency of GPT-3 but also underscores the importance of precise benchmarking to tailor AI capabilities to specific enterprise needs.Â
Through such benchmarks, businesses can truly harness the power of AI to enhance their operations.
Custom GPT Solutions in Academic Research
Academic research often grapples with the challenge of processing vast amounts of data efficiently.Â
Enter CustomGPT solutions, a game-changer for researchers. By leveraging this powerful tool, academics can automate the tedious parts of data analysis, allowing them to focus more on hypothesis testing and less on data wrangling.Â
CustomGPT’s ability to integrate seamlessly with existing academic databases and its support for 92 languages makes it an invaluable asset in global research collaborations.Â
This not only speeds up the research process but also enhances the accuracy and scope of research findings, making breakthroughs faster and more frequent.
Optimizing Performance
Imagine you’ve just tuned up your car for a smoother ride and better fuel efficiency. Now, think of optimizing the performance of language models in a similar way. It’s about fine-tuning these digital powerhouses to ensure they operate at peak efficiency, delivering faster, more accurate responses.Â
In this section, we’ll explore various techniques to enhance the performance of Custom GPT solutions, tackling common challenges that arise as these models scale. From streamlining computational resources to refining model architecture, the goal is clear: to maximize the potential of AI in practical applications.Â
Join us as we delve into the world of performance optimization for language models, where every adjustment can lead to significant improvements.
Techniques for Enhancing Model Efficiency
Optimizing the efficiency of language models like those developed on CustomGPT.ai involves a blend of innovative techniques.Â
One effective approach is pruning, where less important parts of the model are trimmed to speed up processing without sacrificing accuracy. Another technique is quantization, which reduces the precision of the numbers used in the model’s computations, leading to faster and more energy-efficient performance.Â
By applying these methods, CustomGPT.ai ensures that even the most complex models run smoothly and swiftly, making them ideal for real-time applications. These optimizations help in achieving the best balance between performance and resource usage.
Challenges in Scaling Custom Models
Scaling custom models presents unique challenges. Initially, the complexity of integrating these models with existing systems can be daunting. It’s like trying to fit a square peg into a round hole; the alignment requires precision and creativity.Â
Additionally, as the demand on these models increases, maintaining performance without lag becomes critical. This often means upgrading infrastructure, which can be costly and time-consuming.Â
Lastly, the more customized the model, the harder it is to ensure consistency across different environments. Each of these hurdles requires thoughtful planning and robust solutions to overcome.
FAQ
1. What are the key metrics to consider when benchmarking custom GPT solutions?
Key Metrics for Benchmarking Custom GPT Solutions
When benchmarking Custom GPT solutions, several key metrics are essential to assess their performance effectively:
- Accuracy: This measures how well the model’s responses align with expected outcomes. It’s crucial for ensuring the reliability of the AI in practical applications.
- Response Time: Evaluates the speed at which the model provides answers. Faster response times are often critical for user satisfaction, especially in customer service applications.
- Scalability: Assesses the model’s ability to handle increasing loads, which is vital for applications expecting to grow in user numbers or data volume.
- Robustness: This involves testing how well the model performs under various conditions, including handling ambiguous or unexpected inputs without errors.
- Cost-Efficiency: Analyzes the cost of running the model relative to its performance. This is particularly important for businesses looking to optimize operational costs.
By focusing on these metrics, developers and businesses can ensure that their Custom GPT solutions are not only effective but also scalable and cost-efficient.
2. How does CustomGPT.ai ensure the accuracy and reliability of its language models during benchmarking?
CustomGPT.ai ensures the accuracy and reliability of its language models during benchmarking through a rigorous process that integrates both Retrieval Augmented Generation (RAG) technology and continuous improvement efforts.Â
By combining retrieval-based and generative methods, CustomGPT.ai enhances the precision and relevance of its responses. This approach allows for more accurate and context-aware answers, ensuring high-quality AI interactions for users.Â
Additionally, the platform continuously refines its models based on feedback and performance metrics, further bolstering the reliability of the generated content.
3. What are the common challenges faced when scaling custom GPT models, and how can they be addressed?
Common Challenges in Scaling custom GPT models
Scaling custom GPT models can be a complex endeavor, fraught with several challenges that need strategic solutions. Here are some of the most common issues and how they can be effectively addressed:
- Integration Complexity: Custom GPT models often need to be integrated with existing systems, which can vary widely in architecture and technology. This integration can be streamlined using CustomGPT.ai’s no-code visual builder, which simplifies the process and reduces the need for extensive technical expertise.
- Performance Maintenance: As the scale increases, maintaining the performance of the GPT models without experiencing lag or delays is crucial. CustomGPT.ai tackles this by offering optimized model architectures that are specifically designed for scalability, ensuring efficient processing even under heavy loads.
- Cost Management: Scaling up often involves significant infrastructure and operational costs. CustomGPT.ai addresses this by providing a cost-effective platform that minimizes the need for expensive hardware and reduces overall expenditure through its efficient, cloud-based solutions.
- Data Consistency: Ensuring consistency and accuracy of data across different environments is challenging. CustomGPT.ai enhances data handling capabilities, ensuring high-quality, well-structured data is maintained throughout the scaling process.
By addressing these challenges with robust solutions like those offered by CustomGPT.ai, businesses can scale their custom GPT models effectively, ensuring they continue to deliver value at a larger scale.
4. Can CustomGPT.ai be integrated with other tools for enhanced benchmarking capabilities?
Integration of CustomGPT.ai with Other Tools for Enhanced Benchmarking
CustomGPT.ai is designed to be highly compatible and flexible, allowing for seamless integration with a variety of other tools and technologies. This integration capability enhances its benchmarking capabilities significantly. Here’s how CustomGPT.ai can be integrated:
Data Source Integration: CustomGPT.ai supports multi-source data integrations, which means it can pull data from various platforms and databases. This allows for a comprehensive analysis that can be crucial for accurate benchmarking.
API Access: With API access, CustomGPT.ai can connect with other software applications. This is particularly useful for automating benchmark tests and gathering results from different systems in real-time.
Sitemap Integration: This feature enables CustomGPT.ai to easily navigate and index various types of content, which can be beneficial for benchmarking content-driven AI applications.
By leveraging these integration features, users can enhance the benchmarking capabilities of CustomGPT.ai, making it a versatile tool for assessing and improving the performance of language models.
5. What improvements have been made from GPT-3 to GPT-4 in terms of benchmarking performance?
Improvements from GPT-3 to GPT-4 in Benchmarking Performance
The transition from GPT-3 to GPT-4 has marked significant advancements in the benchmarking performance of language models. Here are some of the key improvements:
- Enhanced Accuracy and Nuance: GPT-4 has demonstrated a leap in generating text that more closely mimics human-like patterns and nuances. This improvement is crucial for applications requiring a high degree of linguistic precision and subtlety, enhancing the model’s utility in more complex scenarios.
- Greater Model Efficiency: Despite being only slightly larger than GPT-3, GPT-4 achieves better performance through optimization of model parameters rather than just increasing size. This approach allows for more efficient data processing, reducing computational costs and improving speed without sacrificing output quality.
- Versatility in Applications: GPT-4’s enhanced capabilities extend to a broader range of tasks including language translation and text summarization. This versatility ensures we can effectively benchmark the model across various domains, providing more comprehensive insights into its performance.
These improvements not only signify advancements in the technology but also enhance the practical applicability of GPT-4 in real-world scenarios, making it a superior choice for developers looking to integrate sophisticated language models into their applications.
Conclusion
Imagine you’ve just finished a marathon. You’re exhausted, but there’s a sense of accomplishment that’s hard to beat. That’s the feeling we get as we wrap up our exploration of benchmarking language models with Custom GPT solutions.Â
Throughout this journey, we’ve uncovered the intricacies of language models, from their evolution to the cutting-edge benchmarks that measure their prowess.Â
We’ve seen how tools like CustomGPT.ai not only simplify but also enhance the process, making it accessible to everyone from tech giants to individual innovators. Now, let’s reflect on how future trends in language model benchmarking are poised to reshape the landscape of AI.
Future Trends in Language Model Benchmarking
As we look ahead, the landscape of language model benchmarking is set to evolve dramatically.Â
The focus is shifting from sheer model size to smarter, more efficient architectures. This means future benchmarks will likely emphasize not just accuracy, but how well models handle real-world applications.Â
Expect to see a surge in benchmarks that measure models’ abilities to integrate seamlessly across different platforms and languages, much like CustomGPT.ai does.Â
This evolution will help ensure that the next generation of language models is not only powerful but also practical and versatile in everyday tasks.
- Implementing Financial Compliance with Custom GPT - June 16, 2024
- Enhancing Student Engagement with Custom GPT Solutions - June 16, 2024
- Building Custom GPT for Personalized Fitness Routines - June 16, 2024
